AIOps ushers in a bright future for IT service management (ITSM) operations. It leverages artificial intelligence (AI), machine learning (ML) techniques to automate and enhance various aspects of IT service delivery and management. AIOps platforms pack a big punch because they continuously monitor database performance metrics such as response time, throughput, and resource utilization—automatically flagging deviations from normal behavior. It’s disruptive.
By employing these ML algorithms, platforms can also identify performance trends and proactively recommend optimizations to enhance database efficiency. In particular, the historical performance data and predictive analytics that AIOps can access will help your business forecast potential database failures or performance degradation to minimize downtime and ensure uninterrupted access to critical data.
The ability to do this, uninterrupted, and to respond to the correct notifications, means you finally reduce the number of false positives your ITSM system triggers. This blog post will cover what false positives in ITSM are, their consequences, and two statistical frameworks you can implement within your platforms to reduce the likelihood of false positives from occurring.
False Positives Consequences and Causes in ITSM
False positives in IT Service Management (ITSM) refer to instances where an alert or notification is generated to indicate an issue within the IT infrastructure. And, once investigated, it is determined that no actual problem exists or that the issue is not significant enough to warrant attention. Other than just being noisy and annoying, these false positives have many adverse consequences and causes in ITSM:
- Misconfigured Monitoring Thresholds: Improperly configured monitoring thresholds can trigger false positives. For example, if thresholds for a performance metric like CPU usage is set too aggressively (even normal variations in system behavior can trigger alerts), there can be false positives.
- Transient Issues: Short-lived spikes in network traffic or temporary fluctuations in system performance, can trigger false positives if IT monitoring systems interpret the spikes as indicators of bigger problems. Sometimes the transient issues resolve on their own, but other times they needed the direct assistance of IT teams.
- Monitoring Tool Limitations: Some IT monitoring tools lack the ability to distinguish between normal fluctuations in system performance and genuine issues, which can result in false positives.
- Dependency Failures: False positives can occur due to dependencies between different components of the IT infrastructure that lack the proper correlation and root cause analysis. For example, a failure in one system may trigger alerts for downstream systems not experiencing issues.
- Alarm Fatigue: When alerts consistently fail to reflect genuine issues or require action, IT personnel may become desensitized to alerts (also known as alarm fatigue), which can lead to decreased responsiveness and overlooking genuine IT problems.
- Inadequate Contextual Information: Without sufficient context about the broader IT environment, business impact, or historical performance data, IT teams may struggle to distinguish between genuine issues and transient anomalies—leading to more false positives.
- Manual Errors: False positives can occur from manual errors (human error in configuring monitoring tools) or the misinterpretation of monitoring data by IT staff.
8 ITSM False Positive Threshold Recommendation Best Practices
In the context of false positives for ITSM, threshold recommendations are predetermined limits used to measure the performance of a metric or value. These recommendations can vary depending on the specific context, tools used, and organizational requirements. However, here are some general recommendations, that, when adopted, can effectively reduce false positives in your ITSM platform’s alerting notifications and improve your business’ operational efficiency.
- Baseline What’s Normal: Establish a baseline of normal system behavior before you set a threshold (you need to know where you’re starting from). For example, if a network typically experiences higher traffic volumes on weekdays between 9 AM and 5 PM, this pattern should be incorporated into the baseline, allowing for more accurate differentiation between normal spikes and potential issues outside of these peak hours. The baseline will be specific to your business environment and should consider typical usage patterns, performance metrics, and any known fluctuations—anything that’s considered “normal” and used to measure product performance.
- Tune Alerts and Thresholds: Adjust thresholds for performance metrics (e.g., error rates) based on historical data and observed patterns. Be sure to regularly review and adjust these thresholds over time. TIP: It can be helpful to set a reminder on your calendar or project management tool each quarter to do this.
- Utilize Statistical Analysis: Set thresholds dynamically based on historical data, using standard deviation or z-score analysis. These dynamic thresholds will adjust to performance metric fluctuations, rather than remaining unmoved (i.e., fixed)—reducing false positives caused by temporary spikes or fluctuations.
- Contextualize Alerts: Incorporate contextual information (e.g., what part of the business is this relevant to or who is the task owner of this) into ITSM alerting rules to prioritize system alerts based on their potential impact on critical services or business processes.
- Correlation and Root Cause Analysis: Implement correlation and root cause analysis to identify underlying issues and reduce noise from correlated alerts—this addresses the root cause, not just the symptoms of the issue.
- Feedback Mechanisms: Encourage IT staff to provide feedback on the accuracy and relevance of alerts. Then use their feedback to refine alerting rules, thresholds, and correlation logic over time.
- Automated Remediation: Implement automated remediation actions for known IT issues and/or routine maintenance tasks. This reduces the need for manual intervention and minimizes false positives caused by transient issues. Implementing automated remediation actions can be done by defining clear workflows for automated remediation actions and regularly reviewing them to ensure they remain aligned with your organizational goals.
- Collaboration and Knowledge Sharing: Encourage cross-functional collaboration between all teams within your organization (e.g., operations and development) to foster collaboration and knowledge sharing among all IT personnel.
A number of these practices are directly supported within AIOps platforms. Consult your professional services teams or seek training documentation on how to implement these thresholds and practices.
That said, we want to dig a little deeper on standard deviation and Z-score analysis. Let’s take a look.
The Impacts of Standard Deviation and Z-score Analysis on ITSM False Positives
To further lower your chances of false positive ITSM notifications, standard deviation and z-score analysis can be used to provide a statistical framework for understanding and detecting anomalies in your data within your AIOps platform. Here’s how:
Standard Deviation
The standard deviation is a measure of the variability of a dataset. A standard deviation helps quantify the extent to which individual data points deviate from the average. In the case of AIOps platforms, it’s often used to establish thresholds for normal behavior based on historical data in the system (e.g., response times or network traffic) and determine which deviations that fall outside the expected range may indicate an anomaly or potential issue and require attention. Once thresholds are set, AIOps platforms can leverage these insights gleaned from the standard deviation metrics to trigger alerts and initiate automated remediation actions.
Z-Score Analysis
Z-score analysis is a method for assessing how many standard deviations a data point is from the mean of the other values. The analysis quantifies the distance between an individual data point and the mean (in terms of standard deviations).
A Z-score analysis is used to enhance the granularity of anomaly detection by providing a more precise measure of the statistical significance of deviations for AIOps platforms. A high z-score indicates that a data point is far from the mean— suggesting a significant anomaly. By leveraging a z-score analysis for your AIOps platform, you can prioritize IT alerts and focus your attention on the most critical anomalies presented—allowing you to better differentiate between minor fluctuations and critical issues that require immediate support. It also facilitates a comparative analysis across different datasets and time periods—better enabling your AIOps system to identify patterns and outliers that are emerging issues.
Standard deviation and z-score analysis aren’t everything when it comes to anomaly detection for AIOps platforms. They are tools. They enhance the effectiveness of AIOps predictions by providing statistical measures to quantify and identify anomalies in the data within your system. That said, they can help significantly. By incorporating these techniques into false positive detection algorithms, AIOps platforms can improve the accuracy of predictions—helping your IT team proactively manage your IT infrastructure.
No More False Positives!
As we’ve navigated the twists and turns of reducing false positives within ITSM, it’s clear that marrying statistical rigor with AIOps platforms isn’t just smart—it’s essential. From establishing a solid baseline of “normal” to fine-tuning our approach with standard deviation and z-score analysis, we’ve laid out a roadmap to clearer, more reliable alert systems.
Remember, in the digital ecosystem, false positives are more than just minor annoyances; they’re roadblocks to efficiency and clarity. By applying these frameworks and embracing the power of AIOps, we can transform these obstacles into opportunities for enhanced operational precision and IT harmony. So, let’s reduce false alarms and drive towards a future where every alert is as meaningful as it is actionable—making our IT operations not just buzzword compliant.
2025 Gartner®Market Guide for ITSM Platforms
Get the latest ITSM insights! Explore AI, automation, workflows, and more—plus expert vendor analysis to meet your business goals. Download the report now!
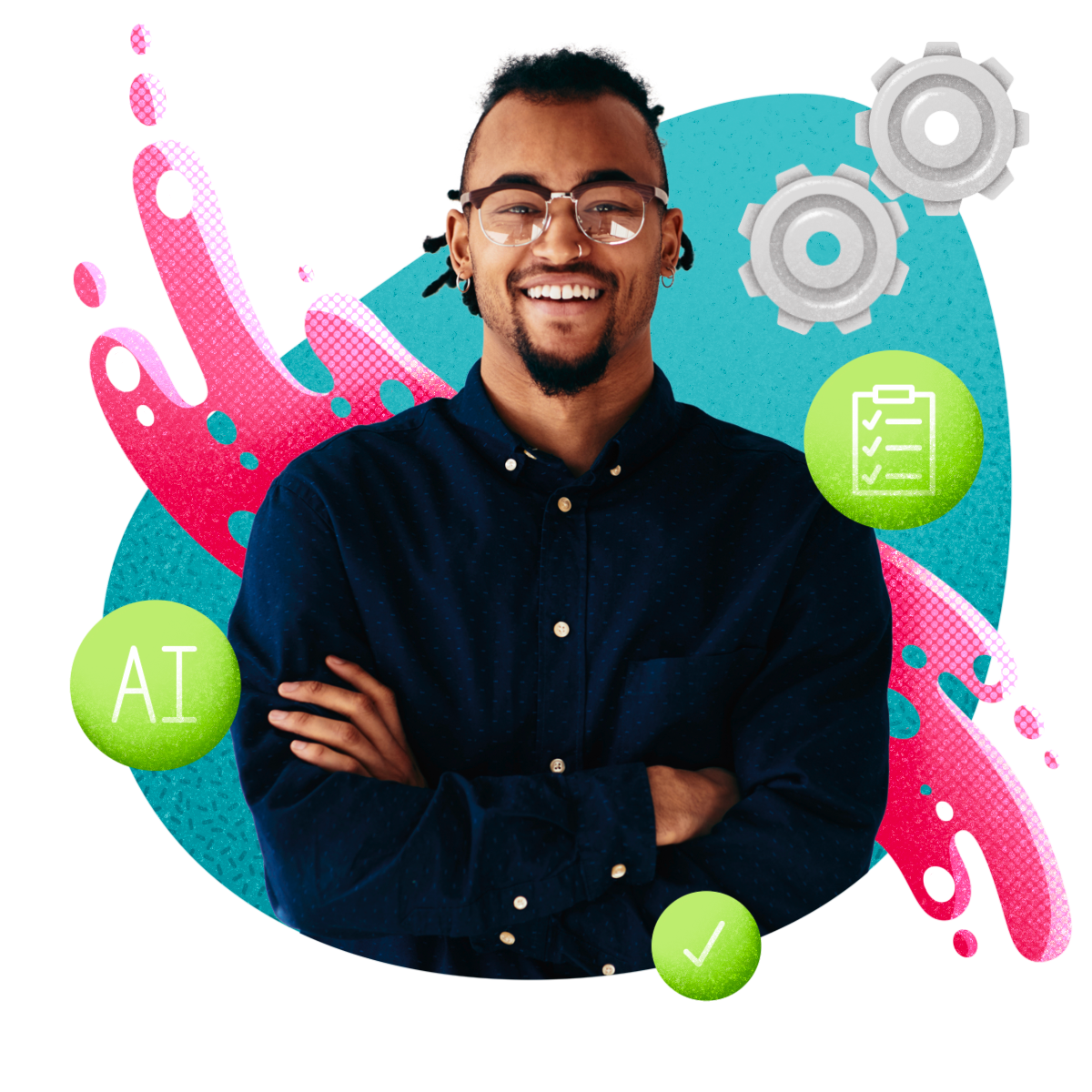